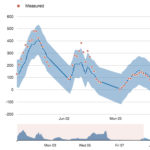
CGBC researchers have launched a new web app that uses statistical modeling and historical data to help predict building energy consumption. The Gaussian Processes Forecasting Tool allows users to upload data, configure features, train/validate a model and make predictions. For a deeper analysis, the app helps more advanced users to explore the impact of certain inputs on output.
Intended to help designers and planners forecast energy consumption of buildings, the app can use inputted data to produce a simulated total energy graph regression throughout the year to see which features have the highest impact. The data can also be configured for different metrics and accuracy, allowing users to explore patterns of energy consumption.
Building energy simulations often have to deal with uncertainty from input parameters, such as the number of occupants, schedule of occupants and occupant habits, as well as simplifications in modeling, whether physics-based or data-driven. Creating more accurate models can be difficult because no single tool can simulate the whole process and it might require more parameters and hence have more parametric uncertainty. With their new app, CGBC researchers have created a more accurate system by analyzing historical data to create a baseline to predict uncertainty in modeling of building data.
Existing tools normalize energy consumption by weather using simple regression methods, while the center utilizes machine learning with the Gaussian process which requires fewer training data points and higher prediction accuracy compared to other machine learning techniques. Additionally, it can evaluate the impact of system control parameters on energy consumption for potential optimization. While it was designed for use with a time-series plot, the app is flexible and can be used to analyze a range of data, such as stock market analysis, power generation and even health changes.